The forex market is known for its high volatility, making volatility forecasting an essential aspect of risk management for traders and investors. Volatility measures the degree of variation in asset prices over time, and accurate volatility forecasting can help market participants make informed decisions about trading strategies and risk exposure.
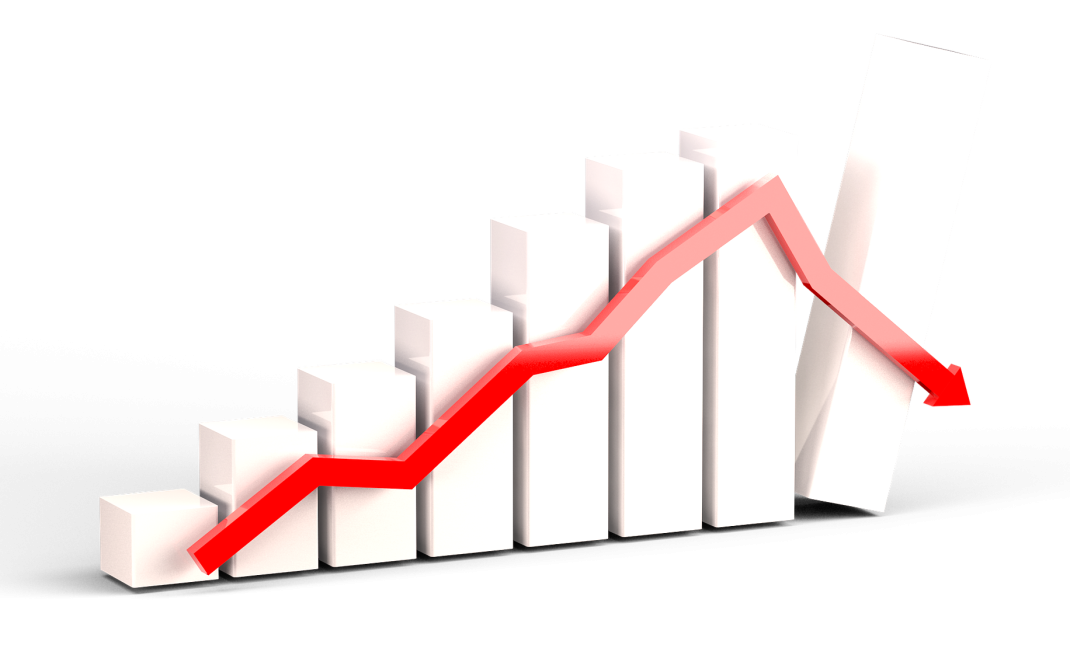
Volatility forecasting is an essential aspect of risk management in financial markets, including forex markets. Generalized Autoregressive Conditional Heteroscedasticity (GARCH) models have become popular tools for volatility forecasting due to their ability to capture the dynamic and time-varying nature of volatility. In this article, we discuss GARCH and Exponential GARCH (EGARCH) models, evaluate model adequacy and diagnostics, and compare them with other models commonly used for volatility forecasting in forex markets.
– How to Develop Winning Forex Trading Strategies: A Step-by-Step Guide
Contents
GARCH and EGARCH Models
Volatility is a key concept in financial markets, and accurate volatility forecasting is important for risk management, portfolio optimization, and trading strategies. One popular method for volatility forecasting is the use of GARCH (Generalized Autoregressive Conditional Heteroskedasticity) models, which allow for the estimation of time-varying volatility based on past returns. The EGARCH (Exponential GARCH) model is a variation of the GARCH model that allows for asymmetric volatility, meaning that positive and negative returns have different impacts on volatility.
GARCH Models
The GARCH model was first introduced by Bollerslev in 1986 as a way to model the conditional variance of financial returns. The model assumes that the variance of the returns is a function of the past squared returns and past variance. The basic GARCH(1,1) model is specified as:
σt^2 = ω + αεt-1^2 + βσt-1^2
where σt^2 is the conditional variance of the returns at time t, ω is a constant, α and β are coefficients that determine the impact of past squared returns and past variance on the current variance, and εt is the standardized error term.
GARCH models have been widely used in financial markets due to their ability to capture volatility clustering, or the tendency of volatility to persist over time. However, GARCH models assume that volatility is symmetric, meaning that positive and negative returns have equal impacts on volatility.
EGARCH Models
The EGARCH model, introduced by Nelson in 1991, is a variation of the GARCH model that allows for asymmetric volatility. The model assumes that the conditional variance of returns is a function of the past squared returns and past variance, as well as the absolute value of past returns. The basic EGARCH(1,1) model is specified as:
log(σt^2) = ω + αεt-1^2 + γεt-1| + βlog(σt-1^2)
where γ is the coefficient that determines the impact of the absolute value of past returns on the current variance.
EGARCH models are useful for capturing the impact of news events and other shocks that may have asymmetric impacts on volatility. The model is particularly useful in financial markets, where negative returns may have a stronger impact on volatility than positive returns.
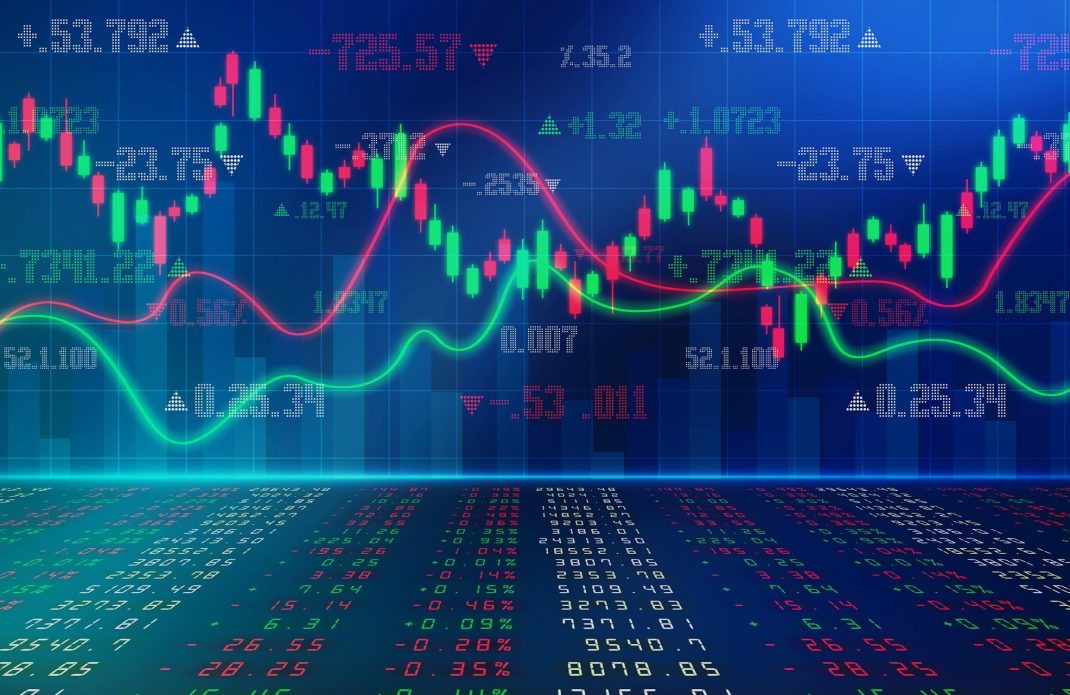
Model Adequacy and Diagnostics
Before using GARCH models for forecasting, it is important to ensure that the model is adequate for the data at hand. Model adequacy tests can be broadly divided into two categories: statistical and graphical tests.
Statistical tests include the Ljung-Box test, the Engle’s ARCH test, and the McLeod-Li test. The Ljung-Box test is used to test for autocorrelation in the residuals, while the Engle’s ARCH test is used to test for the presence of conditional heteroscedasticity. The McLeod-Li test is used to test for non-normality in the standardized residuals.
Graphical tests include residual plots, autocorrelation plots, and partial autocorrelation plots. Residual plots can be used to detect non-linearity, non-constant variance, and outliers in the residuals. Autocorrelation and partial autocorrelation plots can be used to detect the presence of autocorrelation in the residuals.
Model adequacy tests are important because they help to ensure that the GARCH model is appropriate for the data and that the assumptions of the model are not violated.
Model Selection
Once model adequacy has been established, the next step is to select the appropriate GARCH model for volatility forecasting. There are several different types of GARCH models, including GARCH(1,1), EGARCH(1,1), TGARCH(1,1), and IGARCH(1,1), among others.
The selection of the appropriate GARCH model depends on the specific characteristics of the data being analyzed. For example, if the data exhibit leverage effects, then the EGARCH model may be more appropriate than the GARCH model. If the data exhibit asymmetric volatility, then the TGARCH model may be more appropriate than the GARCH model.
– Bitcoin vs Ethereum vs BNB Coin
Model selection can be based on several criteria, including the Akaike Information Criterion (AIC), the Bayesian Information Criterion (BIC), and the Hannan-Quinn Information Criterion (HQIC). These criteria can be used to compare the relative performance of different GARCH models and to select the model that best balances goodness of fit with parsimony.
In conclusion, model adequacy tests and model selection are important steps in the process of using GARCH models for volatility forecasting in forex markets. These steps help to ensure that the GARCH model is appropriate for the data at hand and that the most appropriate model is selected for forecasting purposes. By using GARCH models, traders and investors can make more informed decisions about risk management and can potentially improve their trading strategies.
Comparison with Other Models
Forecasting the volatility of financial assets is a crucial component of risk management and portfolio optimization. In forex markets, where exchange rates between currencies fluctuate rapidly, volatility prediction models are particularly important. In this article, we will compare and contrast the effectiveness of various volatility prediction models in forex markets.
Other models commonly used for volatility forecasting in forex markets include the Autoregressive Integrated Moving Average (ARIMA) model, the Moving Average (MA) model, and the Simple Exponential Smoothing (SES) model. While these models are simpler to estimate and interpret than GARCH models, they often do not capture the time-varying nature of volatility and may produce less accurate forecasts.
Historical Volatility Models
Historical volatility models are the simplest and most straightforward models for predicting volatility. These models use historical price data to estimate future volatility. The most commonly used historical volatility model is the realized volatility model, which calculates volatility as the standard deviation of returns over a given time period. Although historical volatility models are easy to implement, they have a major drawback: they do not take into account changes in the underlying market conditions that can affect volatility.
Implied Volatility Models
Implied volatility models use market prices of options to estimate future volatility. The most widely used implied volatility model is the Black-Scholes model, which assumes that options prices are determined by the volatility of the underlying asset. Although implied volatility models are based on current market prices and can capture changes in market conditions, they are often criticized for being too dependent on assumptions about market efficiency and option pricing.
Stochastic Volatility Models
Stochastic volatility models are a class of models that assume that volatility is itself a random process. These models allow for changes in volatility over time and can capture both the persistence and clustering of volatility. The most commonly used stochastic volatility model is the Heston model, which assumes that volatility follows a mean-reverting process. Stochastic volatility models are more complex than GARCH models, but they can provide more accurate predictions of volatility.
– Application of Microstructural Models in Forex Markets
In conclusion, there are various models for predicting volatility in forex markets, each with its own strengths and weaknesses. Historical volatility models are simple to implement but do not capture changes in market conditions. Implied volatility models are based on market prices but rely heavily on assumptions. GARCH models are widely used and effective, but may not capture all the nuances of volatility. Stochastic volatility models are the most complex but can provide the most accurate predictions. Ultimately, the choice of model depends on the specific needs of the investor or trader and the characteristics of the market being analyzed.